Project - Predictive Delivery Assurance
Defining an innovative quantitative measure of project delivery status and using machine learning to predict when projects are likely to deteriorate, driving proactive interventions and saving millions on overspend and rectification.
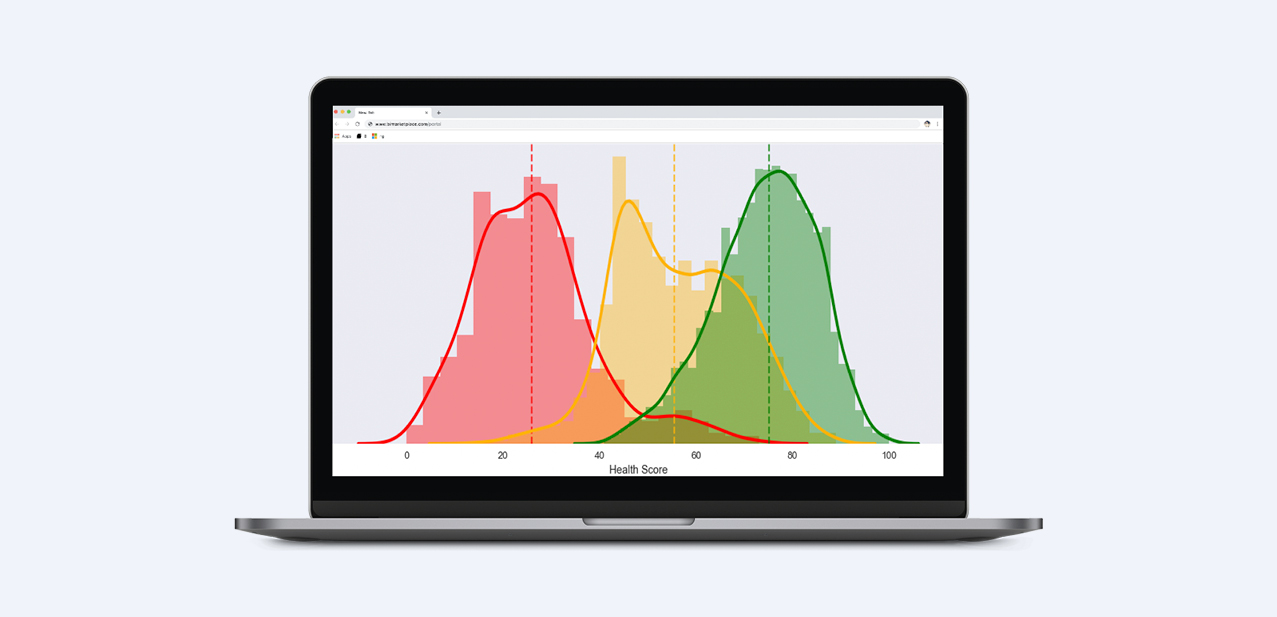
We need to move beyond RAG – it is subjective and coarse – and use data to drive assessment of both current and future delivery status
Summary
The industry project status de-facto of RAG is subjective, biased and coarse. Projects remain stuck in Amber for too long and people are scared to go Red, resulting in delivery inefficiencies.
Our Value Goal was to define an innovative quantitative measure of project delivery status and using machine learning to predict when projects are likely to deteriorate, driving proactive interventions and saving millions on overspend and rectification.
Impact
We created an innovative Health Score that complements RAG-based reporting of the delivery status of a multi-billion change portfolio. Project deterioration predictions are embedded into delivery dashboards to drive more efficient and better informed oversight and assurance.
Proof of Concept created to demonstrate our approach in just 6 weeks leading to request to productionise the models and build up a permanent capability, transforming the way they are transforming change.
Our approach
Combine and enhance data
We brought together data from financial, resource tracking and project reporting systems to form a consolidated view of delivery status. We enhanced the data set by crafting innovative metrics – objective measures of delivery vehicle’s status that are standardized, transformed and input into our machine learning models to produce an evidenced-based Health Score.
Under-reported risk
Health Score was used to uncover delivery risk underreported by RAG and the surfacing of project source system data in this way has driven a marked improvement in data capture and maintenance behaviours.
Predicting a downward trend
We fed historic project performance data into predictive algorithms, applying Natural Language Processing to reveal hidden patterns in PMs’ textual status updates to generate project deterioration predictions that outstripped human forecasts.