How to leverage AI in the fight against financial crime
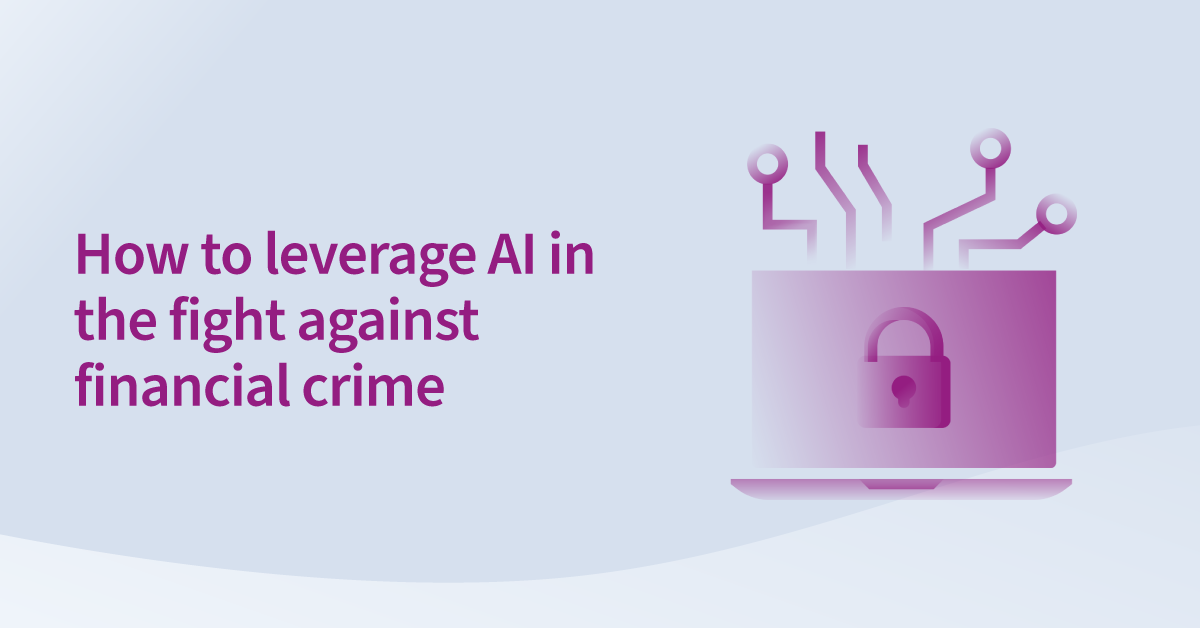
Financial crime has been a real issue over the pandemic. It’s a truism that periods of economic uncertainty create opportunities for criminals to exploit vulnerabilities in banking and payment systems, and since the onset of COVID-19, adoption of digital banking and eCommerce have surged, leading to a massive increase in customers, transactions, and crime.
Perhaps, financial crime in some shape or form will always, but technology can help banks to fight back, regain control, and reduce costs. AI is critical in this effort, but harnessing its power takes more than just top-down decision-making and financial investment. It takes a deep understanding of how people and machines can complement each other.
The scale of the problem
PwC’s 2020 Global Economic Crime Survey found that economic crime reached its highest level in the past 24 months, with 56% of UK businesses surveyed stating that they were impacted by fraud, corruption or other economic crime.
Last year, economic crime reached a record high of $42 billion, with customer fraud, cybercrime, and misappropriation of assets dominating the statistics. Money laundering has exploded by 80% since 2019, and many fraudsters have exploited the pandemic to target individuals with fake prizes and “free” gifts. Add that to the usual challenges of stolen cards, bot attacks, and scamming schemes, and you get a scale of the problem facing banks and their customers.
AI to the rescue?
Most leaders in the banking industry today believe that machine learning is a critical tool for mitigating financial crime. But few understand the complexity of implementing this technology at scale across large financial institutions.
Yes, AI promises great things, but it’s not a panacea. It’s a tool that must integrate with existing systems and compliance processes, providing banks with a flexible, robust and scalable method of triaging and neutralising threats.
To unlock the potential of AI in the fight against financial crime, banks need to leverage the capabilities of machines and people. By applying machine learning to help with triage, firms can make big gains in operational efficiency, while still relying on their existing rule-based models. And firm-level improvements will translate into wider industry growth.
A smarter way to tackle financial crime
A report published by LexisNexis back in 2018 found that worldwide spending on AML and sanctions compliance by financial institutions exceeds $180bn a year. And the FCA estimates the industry spends well over £650m annually on direct staff costs in combating financial crime, before any IT investment or indirect costs. A large proportion of that is spent on inefficient manual investigation due to data constraints and technology challenges.
When working with numbers as large as these, even small improvements to underlying systems can have a meaningful impact on the health of the financial services industry.
Our approach
At Mudano, we believe that resources can be allocated more intelligently and efficiently, by leveraging the power of AI.
Applied machine learning can drive paradigm-shifting improvements in banks’ ability to detect and manage financial crime. Data scientists and self-learning algorithms can help firms use their data to reduce wasted effort through improved identification and triage, and to reduce financial loss through timely and accurate recognition of suspicious activity.
We’re not only at the forefront of machine learning, publishing papers and generating novel techniques – we balance this behavioural science and systems engineering know-how to implement at pace and at scale. We’ve helped clients in a number of ways:
- Improved identification – We use machine learning to inform rules-based alerting systems by applying a basic decision tree model. One client saw a 50% increase in the identification of credit card transaction fraud, and by using clustering techniques on customer demographic data they increased the identification of Authorised Push Payment fraud by 100%.
- Reduced operational costs – We use text analysis to automate heavily manual processes. Extracting themes from text documents was taking our client 4 days a month, just to review a 20% sample. Through text analysis we reduced the process to 40 minutes to review the full data set.
- Improved quality and compliance – We use path analysis to identify root causes. Constructing an events stream of all customer interactions and running path analysis, we enabled our client to identify unknown weaknesses in their process which were triggering issues.
The dynamic interplay of people and machines
Many envisage AI as something that will come to revolutionise banking. But that is already happening. Machine learning is being used across the financial ecosystem, in areas as diverse as new product creation, compliance, and customer service.
AI is also revolutionising crime detection. Operational costs can be reduced through increased model accuracy, explanatory models can keep regulatory risk low, and teams can become more motivated and engaged. This can only happen with buy-in from people on the front line, and that means a focus on data culture and education from the top down.
The future of banking – and the battle against financial crime – relies upon the dynamic interplay of people and machines. And the prize for banks is about more than operational efficiency and cost savings – it’s about gaining and retaining the trust of customers.
The power of AI will hopefully usher in an era where, finally, financial crime doesn’t pay.