Project - Financial Crime Risk Reduction
Cutting edge machine learning reducing false positives and automatically detecting AML risk.
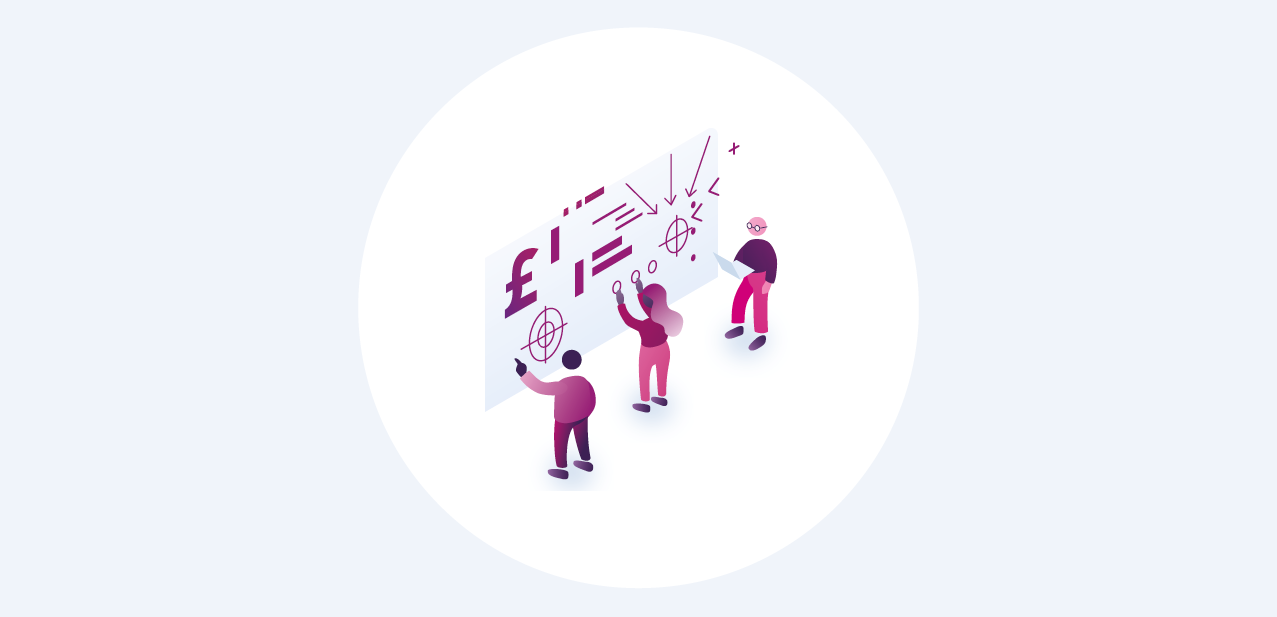
How can we get better at understanding & managing the impact of analytics solutions so that they can be governed in BAU?
Summary
Despite massive investment in software solutions, most financial crime prevention processes are still based on basic, logical rule sets and generate a lot of wasted manual effort. Teams on the ground, although highly specialised, are often facing systemic challenges including:
- High volumes of false positive alerts driving wasted investigations
- Increasingly complex customer behaviour that traditional rule sets are slow to react to, greatly increasing regulatory risk
- Regulatory pressure to demonstrate quality and consistency in investigations
- Poor customer data quality
Our value goal was to drive step-change improvements in the industry’s ability to detect and manage financial crime through Data science and applied Machine Learning.
Impact
- Improved Identification
- Reduced Operational Costs
- Improved Quality & Compliance
Applying Machine Learning algorithms at various stages in the value chain to augment the manual processes reduces existing inefficiencies while increasing insights, ultimately exposing suspicious behaviours and minimising financial crime risk as early as possible.
Our approach
We worked with the client’s in-house data & analytics team to test and improve a customer risk scoring system prior to going live across retail banking and wealth management.
Risk & Operational Impact Assessment
We tested the results of the risk scoring system and developed interactive visualisations that allow stakeholders to run simulations and interrogate the inner workings of the solutions. This allowed making informed decisions ahead of rolling out the solution into the pilot markets and engaging Expert Reviewers and SME validations.
Data Quality Assessment
The programme had been advised to use a strategic data source for customer data which was different to the source used to develop their PoCs. We did a thorough comparative assessment of the data across the two systems. This allowed the project to make informed decisions about any risk implications that need to be accepted and / or resolved ahead of going live in the pilot markets.